High Dimensional Data Analysis
Image
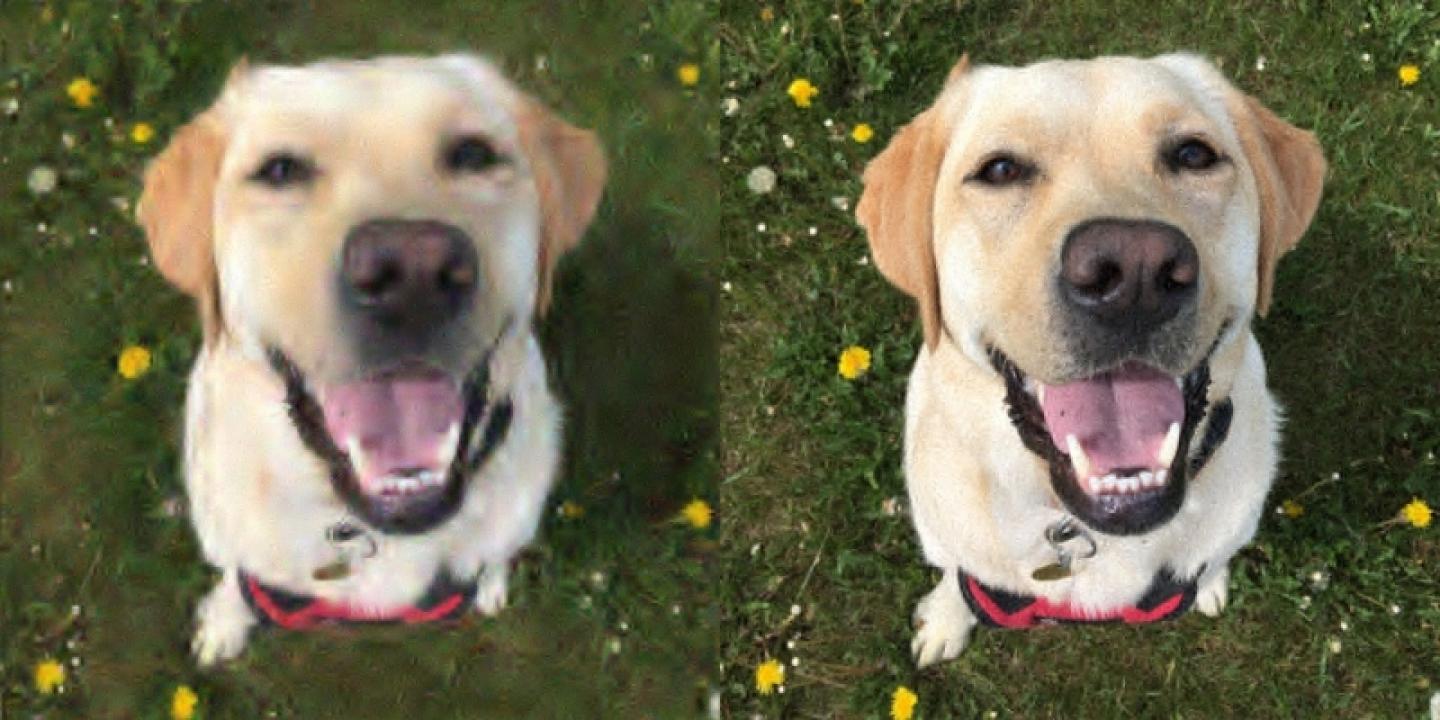
There are fundamental open questions that limit the industrial uptake of ideas from the mathematics of high-dimensional data and their application in practice. These include bridging the gap between the sampling required by theory and what is efficient in practice; translating the theory from analogue to digital; developing algorithms that scale gracefully to big data applications; and implementation of open-source software, which serves as an effective means of technology transfer.
The aim of this CRG to address these questions.Three particular focal areas are:
- Bridging the gap between theory and practice in applications of sparse recovery
- Methods for large-scale optimization
- Deep learning and sampling.
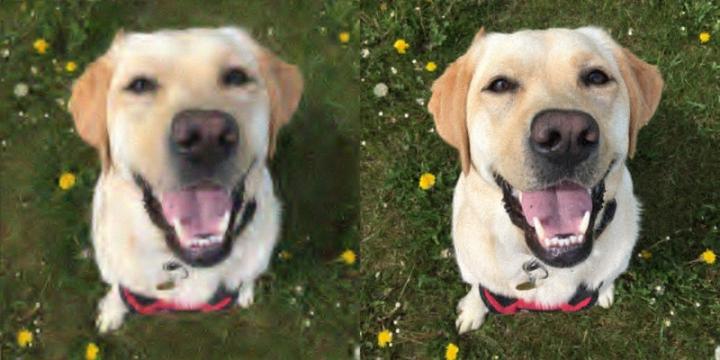